The efficiency of a construction project often hinges on how well resources are allocated. Equipment idling on-site, workers waiting on delayed materials, and unpredictable labor demands can all lead to wasted time and money.
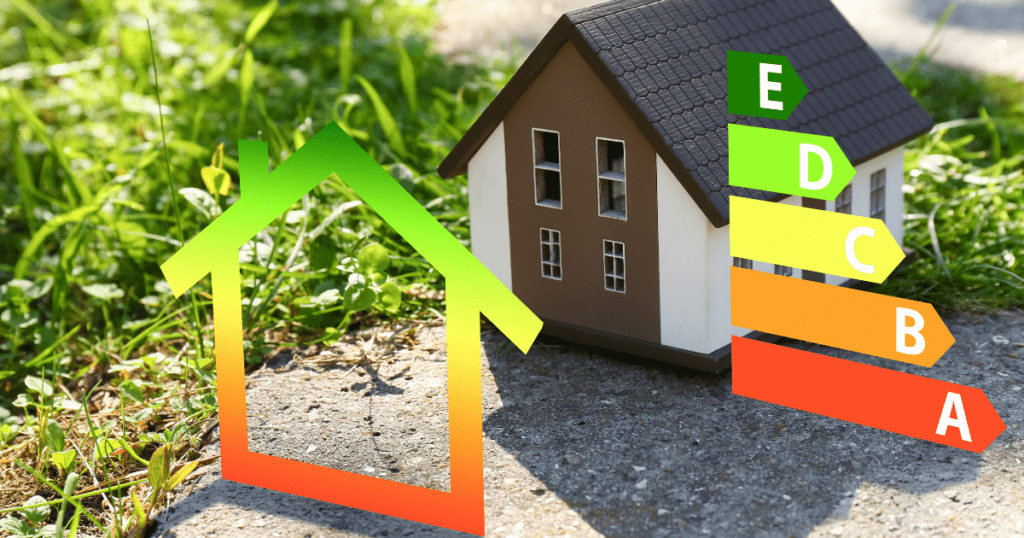
Traditional resource management relies heavily on manual tracking and past experiences, leaving room for inefficiencies. But with machine learning (ML), construction firms are shifting toward intelligent, data-driven decision-making that optimizes resource distribution in real time.
From Guesswork to Precision: The Shift in Resource Allocation
For years, construction managers relied on static schedules, gut instincts, and historical data to allocate materials, equipment, and labor. While these methods worked to an extent, they were prone to human error, delays, and cost overruns. Machine learning introduces an adaptive system that continuously refines its predictions, ensuring resources are placed exactly where and when they’re needed.
By analyzing variables like project scope, worker availability, weather patterns, and supplier performance, ML-powered algorithms can dynamically adjust allocations. This means no more over-ordering of materials, no underutilized heavy machinery, and no unnecessary labor shortages.
Optimizing Workforce Allocation with Machine Learning
Predicting Labor Needs in Real Time
A common challenge in construction is balancing workforce availability with project demands. Assign too many workers to a task, and productivity dips due to overcrowding. Assign too few, and deadlines slip. ML models analyze project timelines, skill requirements, and worker productivity to generate optimized labor schedules.
By leveraging real-time site data, these systems predict peak labor needs and adjust assignments accordingly. For example, if excavation takes less time than expected, ML can redirect surplus workers to another task, preventing downtime.
Reducing Labor Shortages with Smart Scheduling
Machine learning also factors in worker availability, skill levels, and past performance to prevent bottlenecks. If a specific task requires certified electricians, ML ensures their schedules align with the project’s needs, preventing last-minute hiring scrambles. It can even anticipate potential absenteeism trends by analyzing historical patterns, prompting proactive adjustments.
Enhancing Equipment Utilization Through Smart Tracking
Minimizing Idle Time for Machinery
Cranes, bulldozers, and concrete mixers often sit idle on construction sites, incurring unnecessary rental costs. Machine learning optimizes their utilization by analyzing real-time data from IoT-enabled sensors. It predicts when and where equipment will be needed, reducing downtime and unnecessary expenditures.
For example, an ML algorithm might detect that two separate crews scheduled to use the same excavator could stagger their tasks, allowing the machine to be used continuously rather than sitting idle. This kind of precision prevents overbooking or underutilization of expensive machinery.
Preventing Equipment Failures with Predictive Maintenance
Unexpected equipment breakdowns derail schedules and inflate costs. Machine learning enhances preventive maintenance by analyzing sensor data and detecting early warning signs of mechanical failure. By predicting when a machine is likely to fail, ML-driven systems can schedule maintenance at optimal times, reducing unplanned downtime.
Smart Material Distribution for Cost-Effective Construction
Preventing Material Shortages and Overstocking
Ordering too much material strains budgets, while running out of supplies halts progress. ML-driven inventory management systems analyze consumption rates, supplier lead times, and project schedules to ensure materials arrive just in time—minimizing waste and storage costs.
For instance, ML can detect patterns in supply chain disruptions and adjust orders accordingly. If a cement supplier is prone to late deliveries, the algorithm recommends ordering earlier or finding alternative suppliers before shortages impact progress.
Reducing Waste with Precise Forecasting
Material waste is a persistent issue in construction, whether it’s excess concrete, unused lumber, or surplus steel. By analyzing past projects and real-time usage rates, ML systems prevent over-ordering. Additionally, they recommend redistribution strategies, ensuring leftover materials from one project are efficiently repurposed for another.
Integrating Machine Learning into Supply Chain Management
Optimizing Logistics for On-Time Deliveries
Coordinating deliveries across multiple vendors is a logistical puzzle. Machine learning helps predict optimal delivery windows by factoring in traffic patterns, warehouse stock levels, and construction progress. This prevents delays caused by materials arriving too early (leading to storage challenges) or too late (causing work stoppages).
Adjusting for Market Fluctuations
Building material costs fluctuate based on demand, supply chain disruptions, and market trends. ML-driven procurement systems continuously analyze these factors, recommending the best times to buy. If the system detects an upcoming price spike in steel, it may suggest purchasing in advance, saving costs before the increase takes effect.
A Future of Intelligent Resource Allocation
Machine learning is revolutionizing construction resource management, ensuring every worker, machine, and material is used with maximum efficiency. As ML continues to evolve, construction firms will refine their workflows, cut unnecessary costs, and boost productivity with data-driven decision-making.
Also Read:
EzeLogs Quantum: Revolutionizing Construction Levelling
Maximizing Efficiency with BIM VDC: A Guide for Construction Professionals
Quantum Solutions for Construction Project Management
Why BIM Programs are Essential for Modern Construction Projects
Smart Construction: Quantum Scheduling & Resource Planning
Enhancing Risk Mitigation Tracking in Construction Projects