In the complex world of construction, efficiency is not just about finishing on time; it’s about making the most out of every resource—be it manpower, materials, or machinery.
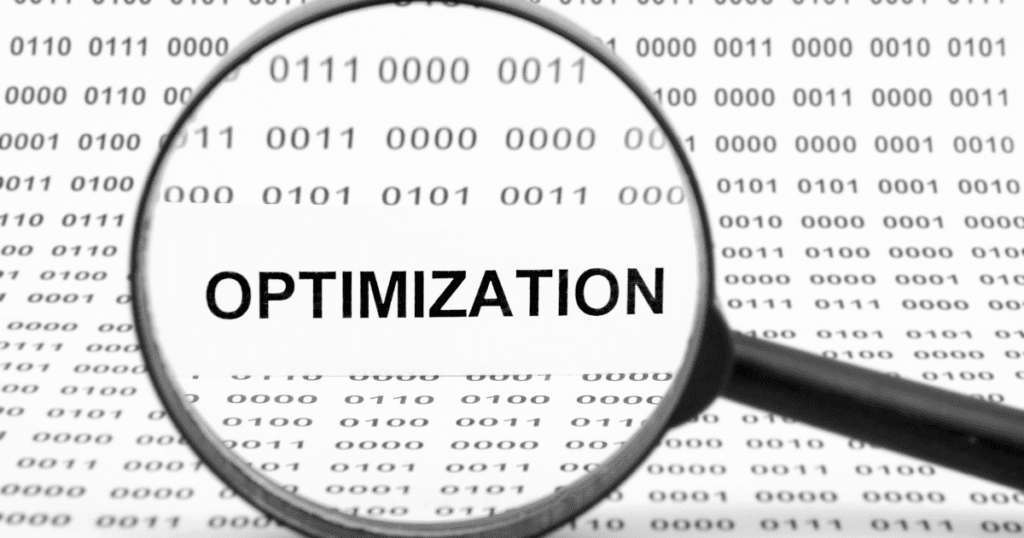
Project managers constantly seek ways to reduce waste, streamline processes, and ensure that all resources are being used to their maximum potential. Traditional methods often involve trial and error, manual tracking, and outdated forecasting techniques that fail to account for real-time fluctuations or unexpected challenges. Enter deep reinforcement learning (DRL), a cutting-edge machine learning technique that is pushing the boundaries of how construction projects are managed and optimized.
Deep reinforcement learning, a subset of artificial intelligence (AI), is revolutionizing the construction industry by enabling more dynamic and data-driven resource management. Unlike conventional systems, which rely on predefined rules and linear approaches, DRL learns from experience, making decisions based on a continuous feedback loop. Through this iterative process, DRL algorithms optimize decision-making, adapt to changing circumstances, and improve over time. This approach holds significant promise for enhancing efficiency in construction projects by optimizing resources in real-time, minimizing costs, and reducing delays.
What is Deep Reinforcement Learning?
Deep reinforcement learning combines two powerful concepts: deep learning and reinforcement learning. Deep learning uses neural networks to process vast amounts of data and recognize patterns, while reinforcement learning enables an agent to make decisions by interacting with an environment and learning from the outcomes of those decisions.
In the context of construction, the “agent” is typically a resource management system, while the “environment” consists of the construction project itself. The agent makes decisions about how to allocate resources, such as workers, machinery, and materials, while learning from the results. If a decision leads to a positive outcome (e.g., reduced waste, faster completion time), the system reinforces that action. Conversely, if a decision results in inefficiency (e.g., a delay due to misallocation), the system adjusts to avoid repeating that mistake in the future.
This learning process is continual, meaning that as the project progresses and more data becomes available, the system becomes increasingly adept at managing resources, improving efficiency, and adapting to real-time conditions.
Enhancing Resource Allocation
One of the most direct applications of DRL in construction is in optimizing resource allocation. Construction projects are dynamic, and the needs for labor, equipment, and materials fluctuate frequently. For example, a project may experience a sudden shortage of a specific material, or weather conditions may delay certain tasks. Traditional resource management systems often rely on fixed schedules and estimations, which can lead to inefficiencies when unexpected changes occur.
DRL, however, is capable of adjusting resource allocation in real-time. By continually assessing the needs of the project, DRL systems can determine the optimal number of workers, machinery, or materials to allocate to each task. If a delay occurs, the system can dynamically reassign resources to maintain the overall flow of the project. Additionally, it can predict potential future shortages or bottlenecks, allowing managers to proactively address issues before they escalate.
For example, if there is a sudden rainstorm that delays outdoor activities, DRL can redirect workers to indoor tasks, ensuring that productivity remains high despite the weather disruption. By considering a wide range of factors—such as worker skill levels, machinery availability, and the stage of the project—DRL provides a more flexible and accurate approach to resource allocation than traditional methods.
Scheduling and Task Management Optimization
In construction projects, the scheduling of tasks is crucial to ensuring efficiency. However, with so many interconnected tasks, delays in one area can lead to a domino effect, causing significant project setbacks. Traditionally, scheduling relied on static models or simple heuristics, which often couldn’t adapt quickly enough to unexpected challenges.
DRL allows for more intelligent task scheduling. By continuously analyzing the project’s progress, DRL can recommend adjustments to the sequence of tasks, ensuring that the most critical tasks are completed first, and that resources are used efficiently. Moreover, the system can adapt to changes as they happen—such as a delay in the delivery of materials or an unexpected surge in labor availability—making real-time adjustments to keep the project on track.
Additionally, DRL algorithms can balance competing priorities, such as managing the limited availability of equipment or optimizing crew schedules. The system can determine the best times for equipment to be utilized, preventing idle machinery and ensuring that it is not overused, which can result in wear and tear.
Improving Material Management and Waste Reduction
Material procurement and waste management are two areas where construction projects often incur significant costs. Overstocking materials can tie up capital and increase storage costs, while understocking can lead to delays. The inefficiency of ordering the wrong quantity or type of materials contributes to both waste and unnecessary expenditures.
DRL systems can significantly improve material management by predicting the exact quantities needed for each phase of the project. By continuously monitoring material consumption and supply levels, DRL can suggest orders for materials at the most cost-effective times, reducing excess inventory and minimizing the risk of running out. Additionally, the system can identify patterns of waste, allowing teams to optimize how materials are used.
For example, if a certain material is being wasted at a higher-than-expected rate, the system can recommend changes in how it is handled or used, helping to curb unnecessary consumption. In turn, this reduces the environmental impact of the project and contributes to sustainability efforts.
Reducing Downtime and Improving Worker Productivity
Worker productivity is another critical factor in maximizing efficiency on construction sites. While construction work is often subject to unpredictable factors, DRL can help minimize downtime by anticipating potential disruptions and allocating labor where it is most needed.
For instance, if a machine breaks down or a specific worker skill is temporarily unavailable, the DRL system can quickly reassign workers to other tasks, keeping the workforce busy and maintaining momentum. Moreover, the system can track worker performance and suggest training or reallocation if certain individuals consistently underperform or if their skill sets are mismatched with the tasks at hand.
By analyzing worker movements and the time required for specific tasks, DRL can also optimize the layout of the construction site, ensuring that workers spend less time moving between areas and are closer to the tools and materials they need. This leads to greater efficiency in day-to-day operations and can significantly improve project timelines.
Scaling to Large-Scale Projects
While the applications of DRL in individual projects are clear, one of the most significant advantages lies in its ability to scale. Large-scale construction projects—such as skyscrapers or infrastructure developments—often involve numerous teams and complex dependencies. Coordinating resources across such large projects manually can be daunting, with hundreds or even thousands of variables to consider.
DRL can handle this complexity by processing vast amounts of data and optimizing the coordination of multiple teams simultaneously. Whether it’s managing subcontractors, materials, equipment, or deadlines, the system can efficiently allocate resources across a massive, multifaceted project. This results in fewer conflicts, reduced delays, and enhanced overall efficiency.
By applying deep reinforcement learning to the management of large-scale projects, construction firms can make smarter, data-driven decisions that improve resource use, reduce costs, and shorten project timelines.
As DRL technology matures, its applications in construction will continue to evolve, offering new opportunities for efficiency and optimization. By leveraging the power of deep reinforcement learning, the construction industry is moving closer to a future where resources are maximized, waste is minimized, and projects are completed faster and more cost-effectively.
Also Read:
EzeLogs Quantum: Revolutionizing Construction Levelling
Maximizing Efficiency with BIM VDC: A Guide for Construction Professionals
Quantum Solutions for Construction Project Management
Why BIM Programs are Essential for Modern Construction Projects
Smart Construction: Quantum Scheduling & Resource Planning
Enhancing Risk Mitigation Tracking in Construction Projects