The Metropolitan Transportation Authority (MTA), the largest public transit authority in the United States, is pushing forward with an ambitious $30 billion capital program aimed at upgrading New York’s aging subway, rail, and bus infrastructure.
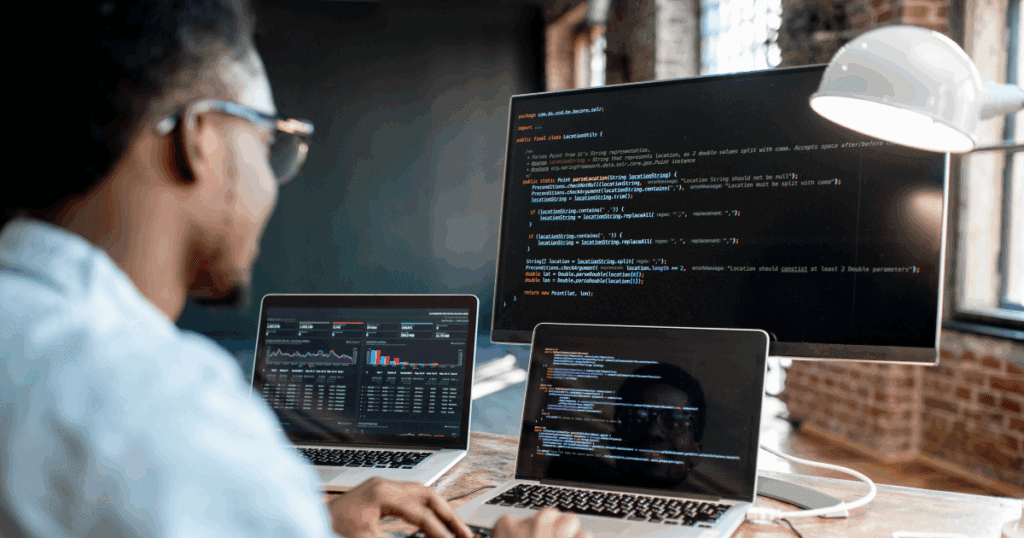
Behind the scenes, a significant transformation is underway—not in track design or tunnel boring, but in project communication. At the center of this shift is artificial intelligence, which is cutting Request for Information (RFI) response times by up to 50%, altering how contractors, consultants, and the MTA itself handle documentation in large-scale construction.
The Weight of the RFI Process in Major Infrastructure
In capital projects like the MTA’s current program, RFIs are a daily occurrence. Contractors submit RFIs when there are ambiguities in the construction drawings, specs, or site conditions. These inquiries require clarification from designers or project managers, and until a response is received, the work often stalls.
Historically, RFI turnaround on infrastructure projects ranges from 10 to 30 days, depending on complexity. Multiply that by thousands of RFIs across hundreds of contracts, and it becomes clear how much delay and cost escalation can stem from this one component.
In the MTA’s case, with over 1,200 active construction contracts across five boroughs, even a modest improvement in communication efficiency translates to millions of dollars in avoided delay costs.
Why AI is a Fit for Construction Admin, Especially in Public Transit
Speed and Pattern Recognition
What AI brings to the RFI process is speed, context, and historical referencing—three things that human reviewers often struggle to maintain consistently when working across hundreds of documents. The technology implemented on MTA projects isn’t speculative or experimental. It’s a practical application of natural language processing (NLP) and machine learning models trained specifically on construction document types: shop drawings, submittals, specs, and architectural renderings.
Matching Past RFIs to New Queries
These AI systems—developed by specialized construction tech firms—automatically tag incoming RFIs, match them to the most relevant contract sections, and suggest likely answers based on precedent from previous similar queries.
For example, if a contractor submits an RFI about a waterproofing detail on a new station platform, the AI might surface a similar issue from a previous borough project, along with the approved response and applicable spec reference.
Real Numbers Behind the Change
In one segment of the MTA’s capital improvement initiative—a $400 million station modernization package—AI-assisted RFI review dropped average response time from 18 days to 9. That metric was logged over a 10-month period involving more than 300 RFIs.
The project team noted a consistent pattern: queries that once required cross-checking multiple design packages and manual document tracing could now be sorted and initially answered within minutes.
Eliminating Redundancy
The AI was also able to flag redundant RFIs—queries already submitted in slightly altered wording—and notify the project engineer to reference an earlier reply. This alone prevented more than 50 duplicate entries from proceeding through the full response workflow, each of which would have consumed hours of admin time.
Integration into Existing Workflows
Built for Compatibility, Not Disruption
Rather than replacing project engineers or architects, the AI tools are being integrated into the project’s existing document management systems, like Procore and Aconex. This allows engineers to receive suggested answers and relevant document references inside their normal review screens.
The MTA’s project delivery team initially piloted the AI tools on two medium-scale station upgrade projects to assess compatibility with union labor documentation protocols and city-mandated public records requirements. Once cleared, the AI was expanded to the broader capital program, including the expansion of Second Avenue Subway Phase 2 and major signal modernization works.
Governance and Data Privacy
These integrations were configured with strict data governance to ensure contract-sensitive materials weren’t used for third-party AI model training, a key concern for public entities under strict regulatory oversight.
Contractor and Consultant Feedback
Better RFI Questions, Faster Answers
From the contractor’s perspective, the AI has made it easier to anticipate how the owner will respond to RFIs. One superintendent noted that the model helped his team craft more precise RFIs that are less likely to be rejected or sent back for clarification. “We’ve learned how to ask better questions because we’re getting immediate feedback on similar issues from other packages,” he said.
Streamlined Review for Consultants
Design consultants also report efficiency gains. With AI auto-sorting incoming RFIs into architectural, structural, mechanical, or electrical categories, discipline-specific engineers can jump straight into their batch, reducing the cross-talk and coordination delays common on multidisciplinary projects.
Even the MTA’s legal and audit departments have found value, using AI summaries to flag potential claims risks tied to delayed RFI responses or vague owner directions, long before they evolve into formal disputes.
AI’s Limitations and Human Oversight
Despite its benefits, the AI is not making final decisions. Each proposed response still requires validation by a licensed professional. The technology is currently viewed as an accelerator, not a replacement.
Where AI Still Falls Short
In some cases, AI-generated recommendations have missed nuances in NYC-specific building code amendments or MTA’s legacy infrastructure standards. For this reason, the rollout includes mandatory human QA checkpoints on all AI-suggested replies.
Also Read:
Safety First: Enhancing Toolbox Talks with AI-Powered Safety Management in Ezelogs
Smart HR for Construction: Boosting Payroll Efficiency with Ezelogs’ AI-Enabled HRM Tools
Compliance Made Easy: How AI-Enabled Certified Payroll in Ezelogs Simplifies Regulatory Reporting
Centralizing Your Data: The Power of Ezelogs’ Product Data Sheet Library for Faster Submittals
Voice-Activated Efficiency: Transforming Construction Management with Ezelogs’