Capital program management for public infrastructure has historically relied on spreadsheets, stakeholder meetings, and a chain of manual processes to track funding, progress, and risk. But as budgets scale into the tens of billions and timelines stretch across decades, some agencies are quietly integrating artificial intelligence to handle complexity at scale. Not for hype—rather for precision, speed, and control.
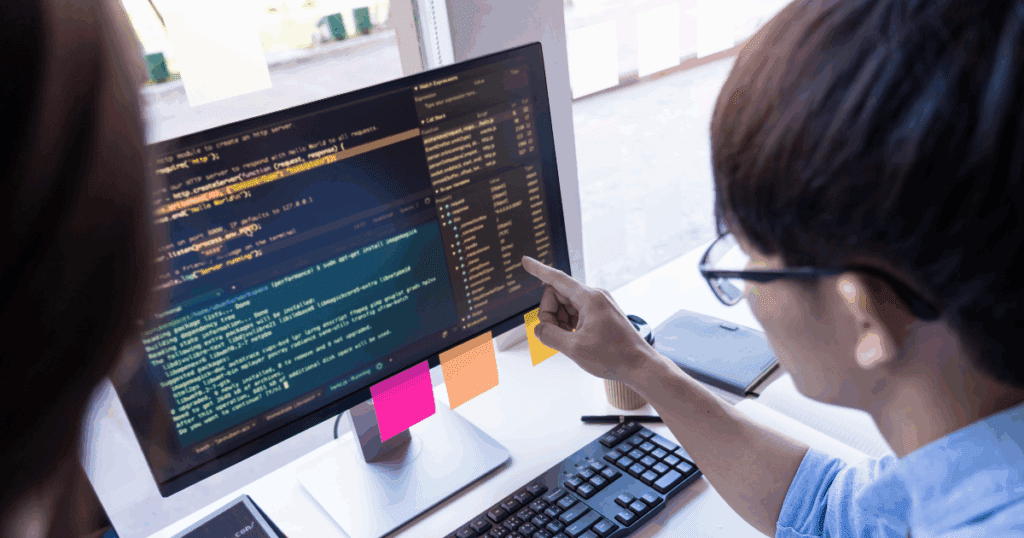
These applications don’t resemble tech startup case studies. They are grounded, tested implementations often buried deep within the workflows of transportation departments, water utilities, and metropolitan authorities managing hundreds of concurrent projects.
Predictive Cash Flow Forecasting at the Massachusetts Bay Transportation Authority (MBTA)
MBTA, overseeing one of the largest transit systems in the U.S., implemented AI models to forecast capital cash flows across more than 300 active projects. Historically, MBTA’s finance team depended on monthly project reports from PMs and baseline schedules in Primavera P6 to estimate cash needs.
The problem: PMs were inconsistent in updates, and delayed field inputs caused forecasting errors. To address this, MBTA integrated an AI layer into their capital program management system. Using historical payment patterns, project type, seasonal burn curves, and contractor performance records, the system started producing forecasts with significantly reduced variance (MBTA Capital Oversight Report, 2025).
One model flagged an upcoming cash overrun three months before it appeared in traditional dashboards. It was due to multiple station accessibility upgrades moving ahead of schedule due to mild winter weather—something the AI detected based on past regional construction trends tied to weather patterns. This allowed MBTA to reallocate available capital and avoid a shortfall.
Prioritization Optimization in the City of Los Angeles Bureau of Engineering
Los Angeles BOE manages over $10 billion in infrastructure work across bridges, street improvements, storm drains, and municipal buildings. The challenge: prioritizing which capital projects should move forward each fiscal cycle given limited funding.
Instead of relying solely on stakeholder lobbying or basic scoring matrices, LA introduced a machine learning model to rank projects based on a composite of impact metrics. Inputs included equity scores (based on socioeconomic data), infrastructure condition ratings, co-location opportunities (e.g., trench sharing with utilities), and estimated job creation (SmartLA 2028 Strategy).
The AI model didn’t make decisions—but it generated ranked scenarios that helped leadership understand the opportunity cost of each prioritization path. In one case, it flagged how delaying a green stormwater infrastructure project in South LA would cause missed federal grant alignment. That insight alone shifted project sequencing and preserved millions in matching funds.
Anomaly Detection at NYC Department of Design and Construction (DDC)
DDC handles more than 500 projects annually, from libraries to firehouses to roadway reconstruction. Within its EPRM (Enterprise Project Risk Management) system, the agency integrated an AI-based anomaly detection module. The intent: catch irregularities in procurement timelines, invoicing, and schedule changes.
The system was trained on five years of historical data, including project typology, schedule structures, invoice approval patterns, and submittal cycle durations. Rather than waiting for PMs to flag issues—or worse, having problems surface during audits—the AI began flagging deviations in real time (NYC AI Strategy).
On one vertical construction project, it detected that steel procurement had deviated from historical lead times despite a seemingly “green” schedule update. Further investigation revealed that the vendor had not yet received the fabrication specs due to a misrouted submittal. The issue was corrected before it turned into a delay.
Document Automation in Washington State Department of Transportation (WSDOT)
WSDOT turned to natural language processing (NLP) to manage document standardization across its statewide capital program. Each project typically generates thousands of documents—change orders, inspection reports, compliance certifications—and each contractor uses slightly different templates and terminology.
Using an AI-enabled document classifier, WSDOT automatically extracts key metadata (project ID, contract value, issue type) from PDFs and emails. A second model maps each document to a predefined workflow path within their project management system, determining whether it requires approval, distribution, or archival (WSDOT ILINX Case Study).
In practice, this system shaved an average of three days off routing time per document and increased visibility into pending approvals across regional offices. It also helped ensure that documentation for federal funding audits was properly cataloged, a significant compliance improvement.
Design Conflict Identification at San Francisco Public Utilities Commission (SFPUC)
In managing multibillion-dollar water infrastructure programs, SFPUC layered AI tools into their BIM workflows. One of the biggest risks in underground utility construction is discovering conflicts between design plans and as-built conditions—leading to change orders and delays.
Using AI-trained algorithms to run clash detection scenarios in Autodesk Navisworks and Bentley platforms, SFPUC was able to proactively identify conflicts between new pipe routing plans and existing fiber optic infrastructure mapped from lidar scans. The system didn’t just detect spatial overlaps; it evaluated likelihood of constructability issues based on similar past clashes and their outcomes (WaterWorld: AI in Infrastructure).
On a major sewer tunnel project, the AI flagged a high-risk crossing zone 40 days before mobilization. The team rerouted the section in the design phase, saving $1.2 million in potential delays and emergency re-engineering.
Schedule Compression Analysis at Illinois Tollway Authority
The Illinois Tollway manages a 15-year, $14 billion capital program. As part of its modernization, it began piloting AI-based schedule compression tools. Unlike traditional critical path methods (CPM), these tools analyze historic project types, resource profiles, and delay patterns to suggest feasible acceleration strategies (Illinois Tollway Reference).
On an interchange reconstruction, the tool identified that switching to weekend closures instead of nightly closures (paired with accelerated cure concrete) would cut 16 calendar days without increasing costs. Project managers had assumed otherwise based on past CPM simulations.
This type of machine-aided insight allowed program leaders to meet aggressive political milestones without compromising safety or quality—an outcome that earned further adoption of AI modeling across the program.
Vendor Risk Scoring in Florida Department of Environmental Protection
With thousands of vendors applying for infrastructure grants or contracts annually, the Florida DEP implemented an AI model to score vendor risk. This wasn’t just based on past performance. The model looked at bonding history, litigation records, geographic concentration, subcontractor history, and even OSHA citation patterns.
By using publicly available datasets and internal performance tracking, the AI generated a risk index for each vendor. High-risk vendors were flagged for secondary review, and lower-risk vendors were fast-tracked for prequalification. It reduced the average vendor review cycle time by 22% while strengthening due diligence in federal compliance programs (Florida DEP Site Priority Guidance).
Also Read:
Safety First: Enhancing Toolbox Talks with AI-Powered Safety Management in Ezelogs
Smart HR for Construction: Boosting Payroll Efficiency with Ezelogs’ AI-Enabled HRM Tools
Compliance Made Easy: How AI-Enabled Certified Payroll in Ezelogs Simplifies Regulatory Reporting
Centralizing Your Data: The Power of Ezelogs’ Product Data Sheet Library for Faster Submittals
Voice-Activated Efficiency: Transforming Construction Management with Ezelogs’